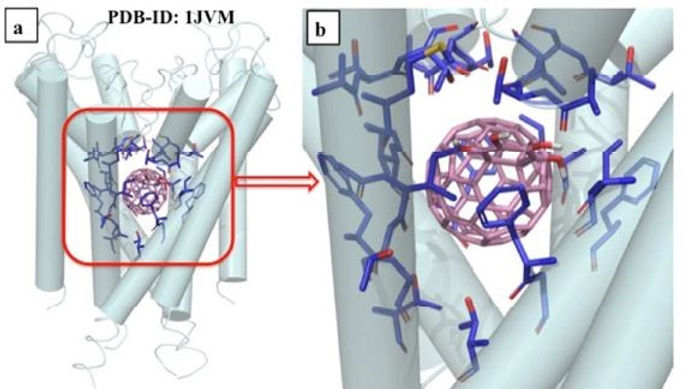
Modelling and prediction of drug induced toxicity to human employing QSAR modeling
I have modelled pharmaceuticals adverse drug reactions (ADR) to humans employing multiple cheminformatics approaches like QSTR, pharmacophore and docking. My developed QSTR models helped to identify the responsible structural features for drug toxicity to human and the major mechanistic hypothesis for toxicity. Pharmacophore helped to compute the scaffolds for toxicity in specific drug’s chemical structure followed by docking study to identify the interaction of those drugs to the receptor for toxic effects. I have modelled multiple less explored ADRs to human which are not modelled previously by any researchers. That’s why my developed models are very much important toxicity data repository for pharmaceuticals industries. I have developed reliable and mechanistically interpretable models for systemic toxicity, milk/plasma concentration ratios, hERG potassium channel blocking actions, eye irritation, skin sensitization and carcinogenicity. Employing my QSTR models, industries can check above mentioned toxicities for newly developed drug molecules. For data gap filling purpose, I have employed my models to predict DrugBank database which is an important source of marketed as well as drugs under clinical trials to predict above mentioned toxicity. The predicted toxicity of drugs under clinical trial can help to decide for the approval of those drug candidates.
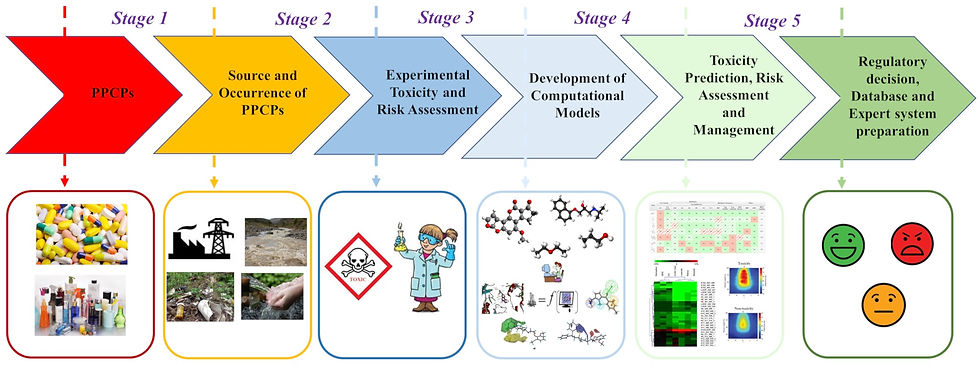
Ecotoxicological assessment of chemicals, pharmaceuticals and personal care products using predictive toxicology approaches with special interest to QSAR modeling
I have developed multiple quantitative structure-toxicity relationship (QSTR) models which help to understand the responsible structural features and physicochemical properties for the toxicity of the studied chemicals and PPCPs covering mammals to bacteria. The developed QSTR models helped in designing of chemicals and PPCPs by predicting possible toxicity values for the studied species thus serving us to decide on making expenditure of synthesis and further testing of comparably less number of candidates. My model is capable for screening and prediction of untested chemicals and PPCPs which are already in the ecosystem. As prior to 2007 there was no requirement of toxicity testing related to environment before approval of chemicals to the market, thus there is huge number of compounds exist in the market which have no toxicity data at al. Thus, my developed models are important for not only toxicity prediction but also for risk assessment analysis. As in silico models are very much important now a day for US EPA rules guidelines and decision making for risk analysis of untested and new chemicals, my models are very important concerning this aspect. For fast, animal free and economical toxicity prediction, industries, government regulatory authorities throughout the world relying majorly on these computer models. [Figure Copyright: Kar et al. Green Chem., 2020,22, 1458-1516]
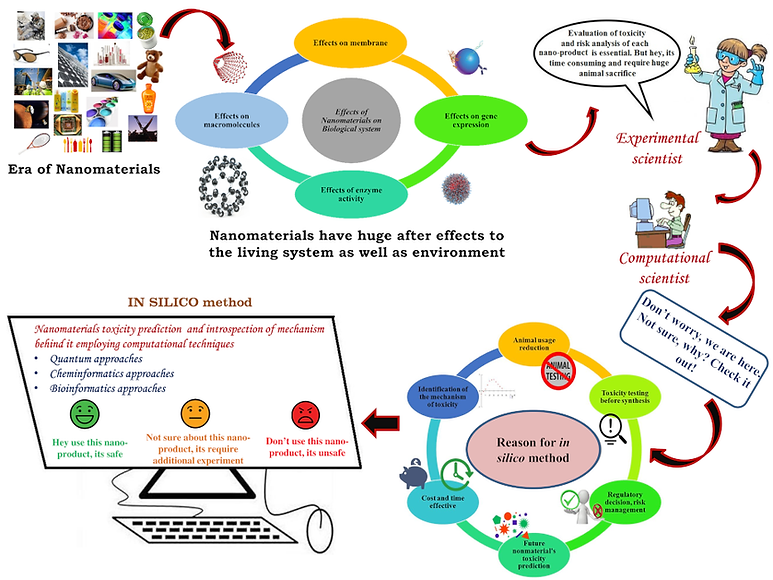
Advancement of QSAR approach for prediction of toxicity of nanomaterials followed by applications
The application of cheminformatics in the risk assessment of metal oxide nanoparticles (MNPs) and data gap filling has found profound usability. Among cheminformatics tools, quantitative structure-toxicity relationship (QSTR) tool is one of the front runners to develop mechanistically interpretable models which can predict toxicity along with identify the responsible physicochemical properties for toxicity of MNPs to specific species. Due to complexity of MNPs size and structure variations, development of new advanced methods is very much significant for toxicity modeling and toxicity prediction purpose. I have developed new descriptors to model toxicity of MNPs employing periodic table which can be computed in no time. Before that, time consuming experimental parameters and quantum information are utilized to model the toxicity. My discovered periodic table-based descriptors helped to develop and predict the toxicity of new and untested MNPs in no time as the computation of those descriptors are easy and fast. Further, I have developed a new method of nano-quantitative toxicity-toxicity relationship (nano-QTTR) which can be used to extrapolate toxicity data of MNPs from one species to another species when experimental toxicity data of one species is present and another one’s absent. The technique is very much significant considering data gap filling and utilizing of experimental data for one species to another one in time. Along with development of new descriptor and method for nanomaterial modeling, I have modeled MNPs toxicity to three different species E. coli (Bacteria), HaCaT (Human cell lines) and Zebrafish which spanning over all compartment of environment so that regulatory bodies and nanomaterial industries can have sufficient knowledge about MNPs toxicity and reason behind it. Additionally, cellular uptake of MNPs is modeled by me for three diverse cell lines PaCa2 human pancreatic cancer cells, U937 macrophage cell lines, resting and activated primary human macrophages, and HUVEC human umbilical vein endothelial cells. Based on the cellular uptake modeling, I have developed another new method called quantitative inter cell line uptake specificity (QICLUS) modeling to identify the physicochemical properties, as well as majorly structural fragments responsible for cellular uptake differences between two specific cell lines which can categorize different toxicity reason of specific MNPs to two different species.
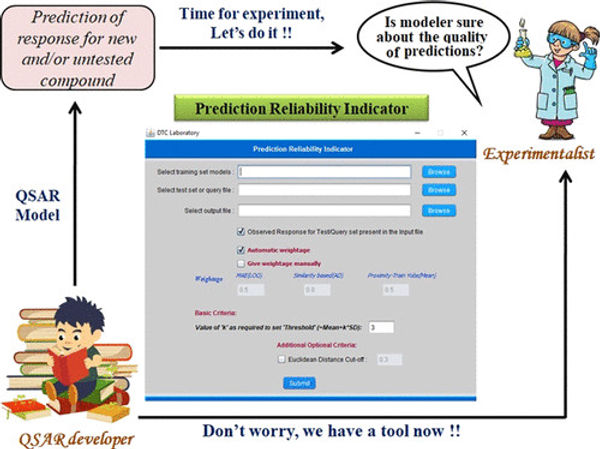
Development of new validation tools and metrics for QSAR approach
Validation strategies seek to explore the reliability of the developed computational models. I am part of the team which developed much more rigorous and stringent validation parameter known as rm2 metric followed by developed software to calculate the metric. My developed metric depends mainly on the difference between experimental and predicted data and convey more accurate information regarding the quality of prediction. Thus, its strictly judge the ability of a developed quantitative structure-activity relationship (QSAR) model to predict the response of untested molecules than the existing classical validation metrics. I am part of the team which have further developed rm2(rank) metric where prediction quality can be prioritized based on ranking system to give proper idea about prediction and classify a set of molecules based on response data. All rm2 metrics were employed extensively to check the quality of the developed models worldwide by different research groups and now this is one of the established validation metrics known among the cheminformatics scientists.
​
In another study, to improve the prediction quality, I am part of the team which have discovered “intelligent” consensus modeling approach, taking into consideration the aspect of interpretation of models. Therefore, consensus models not only improve the predictability, but also, they have the capability for complete interpretation based on the identified responses from all developed models.
To classify the prediction quality, I am part of the team which have developed another method and implemented into a software that is an user friendly tool named “Prediction Reliability Indicator” to check the prediction reliability score, followed by classification of predictions in term of “good”, “moderate”, “bad” along with details about applicability domain (AD) information and QSAR statistical parameters. [Figure Copyright: Roy et al. ACS Omega 2018, 3, 9, 11392–11406]

Design, prediction and optoelectronic property evaluation of power conversion efficient solar cell systems
I have developed first comprehensive QSPR models, followed by screening/designing of new acceptors for PSCs and dye sensitizers for DSSCs. Based on the developed QSPR models, I have developed Fullerene derivatives as acceptor for PSC which theoretically showed 12.11 % power conversion efficiency (PCE) which provided increment in PCE compared to existing FDs for PSCs. In case of DSSCs, I have designed 7 advanced dyes each for three chemical classes namely tetrahydroquinoline (THQ), N,N′-dialkylaniline (NDI) and indoline (IND) classes. The potentially best dye among the developed 7 dyes for each classes, reported PCE value of 18.88 (THQ9), 19.24 (NDI6), and 13.87 (IND10) predicting 130%, 183%, and 46% increment in PCE when compared to existing dyes. Along with the elevated PCE value prediction, electrochemical parameters, absorption spectra and contour plots of HOMO and LUMO of each lead dyes helped to check required electrochemical environment for fast electron transfer rate in the composite PSCs and DSSCs system to support their better PCE. The encouraging outcomes of the performed studies allow us selecting new efficient FD as acceptor for PSCs and dye sensitizers for DSSCs to obtain higher photo-to-electron conversion efficiency. [Copyright: Kar et al. npj Computational Materials volume 3, 22 (2017)